Translation memory? Even more hyped-up memory? Supercool MT-supported memory? Don't buy that loaf: showing exact matches, "hyping up" memories is easy and an old school thing. Been there, done that. A real CAT tool offers better and better suggestions from a range of exciting resources with a range of exciting tools.
Here’s a cliché: a translation tool aspires to make the labor of translation more productive – to help translators and organizations complete translations in minimum time and in maximum quality. Translation tools employ various feats and tricks to boost the number of words we can translate in a day.
Translation environments are traditionally built around a translation memory, and when we must gauge how much we need to work on a translation, we usually count the different kinds of matches from the translation memory, and seldom count anything else.
A good translation tool helps a lot even when there’s no match
But it’s no longer enough to just remember translations. (It never was, actually.) The time pressure of translation jobs is always getting worse, and translation tools are expected to offer help even when there is no match from the translation memory.
The trick is not to find the same sentence – or a slightly different one. The trick is to offer meaningful help even when there is no good match from the translation memory.
(It’s always possible to tie in a machine translation program, and use hints from it when all else fails. But machine translation is no oracle, either – and in many cases, it’s an overkill even: much simpler tools like replacing terms or numbers can mean a lot.)
As a result, a suggestion in a translation tool can come from many different resources. In memoQ, it’s translation memories, LiveDocs corpora, Muses, term bases, auto-translation rules, machine translation. From translation memories and LiveDocs corpora, memoQ can give you fragments, concordance – and if you throw in a term base, memoQ can patch your TM match by updating a term or two in the suggestion. (Actually, memoQ can also use fragments from the translation memory for that.)
Complicated? It doesn’t have to be. For starters, as a translator, you don’t have to look out for all of these when you translate. You just receive a suggestion, and enjoy the benefits. Which will be a mix of the outputs of all of the above.
Those who know Hungarian will see that this suggestion is not a good translation but a good start to produce one. Note that memoQ highlighted only one term in the source text, but replaced much more in the target cell. The underlying secrets will soon be revealed.
A real-life project: translating manuals
In the memoQ production team, I’m responsible for documentation and localization. (And I’m up to great things, but that’s not the point today.) Recently, we had to update the translation of the memoQ interface to all the languages we support.
We eat our own dog food: we localize memoQ using memoQ. I’m running a translation organization inside Kilgray. I take orders from various departments, and I work with vendors – freelancers and translation companies – to fulfil them. To get a taste of the work of a linguist, I sometimes translate a bit, mostly into Hungarian. The last but one example was the 8,000 words of new strings that were missing from the Hungarian interface of memoQ.
I usually take memoQ’s hints for granted, and only watch for the new features, or problems that need reporting – sometimes I’m the first to use a new feature in production. But after I listened to another tool producer at a conference, I started noticing all the coolness memoQ gives me:
Even when there isn’t a match from the translation memory, I often receive a suggestion with many useful parts. Out of tradition, memoQ still shows the list of everything it found, but I don’t have to look for the list. Either the relevant parts of the source text are already replaced, or I get the hints in predictive typing, right there in the target cell.
Just yesterday, I was translating the two basic memoQ manuals into Hungarian. Now English into Hungarian is a very tricky language pair. If you want to do machine translation from or into Hungarian, you need to fight your way through all the suffixes and compounds. In most European (Indo-European) languages, there are things you express with prepositions and possessive structures of three or more words. In Hungarian, the same thing will often be one word. If you don’t use linguistic processing (linguistic stemming as a minimum), you’ll need several times more Hungarian words to train a MT engine to make it any useful.
Preparations
Keeping this in mind, here’s how I prepared the project to translate our English manuals into Hungarian:
I’ve added the translation memory of the user interface strings. User interface strings are pieces of text you see anywhere in the memoQ window.
I’ve added the official Kilgray term base where we store external and internal terminology as well.
I had to realize there was no translation memory of Hungarian user manuals. The last time these manuals were translated into Hungarian was for version 4.5, in 2011. Now we are at version 7.8.150+. So, I’ve fired up a LiveDocs corpus, and just threw in the two manuals that were written for memoQ 4.5, along with their translations. When you throw a pair of documents into a LiveDocs corpus, memoQ aligns their contents automatically at the sentence level. I didn’t review the alignment – just started to use the matches from the corpus.
From the translation memory and the LiveDocs corpus, I’ve trained a Muse. In memoQ, a Muse is a resource – a statistical database – that gives hints for predictive typing. Computing from the source text and the translation that was already typed, it will guess the next word or expression. This is similar to the hints you get from Google when you start typing a search expression – but this time, if you pick the right resources to train your Muse, they will be very relevant to your translation.
In memoQ, you don’t have to collect a minimum number of words or segments before you can train a Muse. In my example, it was altogether 24,000 segments, roughly 200,000 words.
Disclaimer: Training a Muse is all statistics. No linguistic processing is involved.
Putting the project together took 5-6 minutes (I haven’t timed it). After finishing the first document, I’ve retrained the Muse so that predictive hints take into account the latest translations, too. That took two more minutes in the middle of the project.
Fragments and fortune-telling
Like I wrote, the feat isn’t when the exact same sentence turns up again. A translation tool reveals its best secrets when there’s nothing from the translation memory, at least not from the entire segment. I’ve collected some examples from this last job:
Let’s start with the hint you’ve already seen:
Quite a few words were replaced, so that I don’t have to type a brand new translation. Luckily, the word order is more or less OK, so we can just go and adjust the hint. Here’s the same segment while in the works:
See the suggestion for predictive typing? It’s not a term, a number, an abbreviation, or any other ’placeable’: it’s a word that often follows the previous one – it’s coming right from the Muse. I just press Tab and go on to replace ’is about’ with ’körülbelül’.
Let’s see where all these replacements come from. Like I wrote, you don’t have to care about it – but if you do, memoQ gives you a list on the right. That list is called Translation Results. For this example, here it is:
There are no red items in the list. This means that the translation memory and the LiveDocs corpus had nothing to contribute. Or did they? We’ll find out in a moment.
The first row is a term base match (somehow it was cropped from the image). It says we translate ’memoQ’ as ’memoQ’. It could be part of a list of non-translatable items (that’s another type of resource that memoQ knows about), but quite understandably, the term ’memoQ’ happens to be an important item in the Kilgray term base.
The second row warns of a forbidden term. This will not be replaced or inserted anywhere. It tells me never to translate ’memoQ’ with a capital ’M’. If I do, I’ll receive a warning from the automatic quality assurance (QA) module.
The third row is a 'fragment-assembled' suggestion. You can safely skip it: this was the suggestion that memoQ originally inserted in the target cell.
The rest of the hints are orange. These come from the translation memory or the LiveDocs corpus, but they don’t span the entire source segment. These are all translations of user interface strings, which happen to occur inside the sentence I’m to translate. So the translation memories have something to contribute after all.
The orange hits are called fragment matches. When a segment has no match from the translation memory, but there are term hits and fragment matches, memoQ will replace the matching parts and offer a stitched-together translation. In most cases, you can efficiently work them into a proper translation, unless the word order must be very different.
Here’s another example:
Concordance with translations
This suggestion needs a bit more work than the previous one. I brought it up because the list of orange hits has items that don’t have a translation:
When an orange hit has a translation, you know there is an entire unit in the translation memory or in the LiveDocs corpus with that source text. But when there isn’t a translation, you know it’s a fragment of the source text in a unit of a translation memory or a LiveDocs corpus.
In other words, memoQ runs concordance automatically for you, and brings up the expressions that occur several times. In the example, ’you need to’ and ’for the day’ are such examples.
You can fish for translations of these: just double-click the orange box. Obviously, memoQ won’t insert a translation this time. Instead, the Concordance window appears:
The Concordance window gives you the context of the expression from the translation memories and the LiveDocs corpora (at once from all translation memories and LiveDocs corpora that you use in your project). But it does much more: look at the highlighted words. The English expression is highlighted in orange – and in the translations, memoQ tries to find the translation. That’s the green highlight. memoQ’s choice in all the examples is ’kell’, which happens to be correct. This time there are only 60 hits, which is nowhere near the amount you would need for proper statistical processing.
The Muse, by the way
Note that the Muse will most probably know about this.
As it happens, the Muse is most useful when there is nothing, not a match from the translation memory or from LiveDocs, no term matches or fragment finds. This means you need to type the translation from scratch. This is when predictive typing will give you a lot of goodies:
Normally, predictive typing will offer up to 5 hints (including properly suffixed verbs!). If the item you need is at the end of the list, just press the up arrow, and you will get there.
The Muse also knows about phrases of common discourse:
This is a translation for ’In this case’.
Patching a good match to make it an exact one
Finally, let’s see what happens when there actually is a pretty good match for the source segment – but there is one term or fragment that’s different:
For the segment ’Replaces text in the translation grid’, memoQ will find ’Finds text in the translation grid’. The match I received was automatically ’patched’ by memoQ: the canned translation had the word ’keresése’, but memoQ automatically replaced it with ’cseréje’. How was that possible?
On the one hand, the term base contains ’Finds’, translating into ’Keresése’. Now these are not dictionary words, not the forms you would actually include in a term base. In memoQ, that entry looks like this:
The English term is ’find|s’ that indicates that the stem is ’find’ but we allow the ’s’ – in fact, any suffix – at the end. In Hungarian, the term is ’keresés|e’, where the stem is ’keresés’, but we allow the ’e’ – in fact, any suffix – at the end.
For those who know Hungarian, ’keresés’ is in fact a noun, but in user interface, Hungarian translations often ’nominalize’ English verbs, so this is a perfectly appropriate entry in a term base.
This helps memoQ identify the term that changed. But it still hasn’t got the translation for the new term. For that, there’s another entry in the term base, translating ’Replaces’ into ’Cseréje’:
Again, notice the pipe (|) characters in the word forms, indicating the end of the stem of the word. If this is there, memoQ will try to match the stem only, and won’t worry if the suffix isn’t the same as in the text.
So, from the translation memory match, memoQ knows that the difference is the word ’Replaces’. From the first term base entry, it knows that the translation of the original word ’Finds’ is ’keresése’ - that needs to be found in the canned translation. memoQ needs to know this so that it can find the words that needs to be replaced.
Finally, from the second term base entry, memoQ knows that the translation of the new term is ’cseréje’, which needs to be used instead of ’keresése’ in the new translation.
Complicated? No. Maybe in the description, but as far as natural language processing goes, this is a no-brainer.
The morale of the story? If a translation tool doesn’t find an exact match for a piece of text, it can still do a lot of simple things and offer suggestions that can be as useful – or, at times, more useful – than a machine-translated suggestion. We have just dissected these suggestions – to show that memoQ indeed goes many extra miles to help a translator when there is no match or good machine translation.
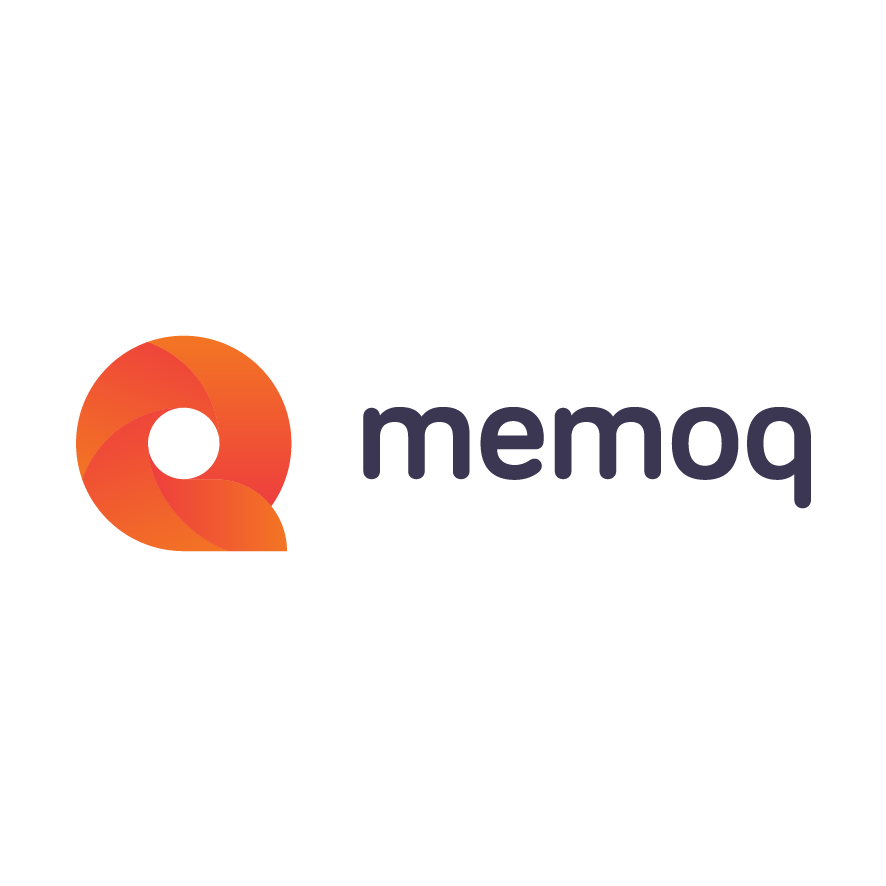
memoQ
memoQ is among the world's leading translation management systems. The favorite productivity tool for translation professionals around the globe.