It seems that more and more translators and translation providers realize the opportunities Machine Translation has to offer. This has been reflected in our recent mini survey and various reader responses too.
So how good is Machine Translation for us? Our guest author is heading the company producing Slate Desktop, an innovative desktop MT solution. His insight is valuable: how does someone coming from the MT scene see the role, purpose and value of machine translation in our daily work?
Instead of telling stories about machine translation's blunders and reality you probably already know, I’ll share some things I learned as I came to the world of machine translation (MT). In the process, I hope you’ll gain a new perspective about one of memoQ’s sexiest features, machine translation plugins.
I started my career as a US Government employee. I wasn’t a computational linguist or a translator. I was a technician and project manager for speech technology projects. Starting in the early days of personal computers, I worked side-by-side with translators as colleagues. The translators were the final link in critical project deliveries. Fond memories of those rewarding times keep me going in this business.
By 2002, I’d moved to the private sector as a localization rookie. I assumed translation software created translations. How foolish of me! A small LSP’s CEO taught me that translation software managed complex translation projects, that MT software created translation suggestions, and that a translator’s work was too complicated for MT to replace professionals.
First Generation RbMT
At that time, the market-leading MT software was Systran. They pioneered the first generation of MT technology called rules-based machine translation (RbMT). Systran sold desktop applications and licensed engines that powered websites like babelfish.com. The market consensus was RbMT rarely created correct results, i.e. translations that could be published without editing.
The industry developed business process to compensate for RbMT’s flaws. Complex error metrics helped computational linguists improve rules and update dictionaries. Consultants taught authors and translators to compromise their professional judgments so RbMT could produce its best results. With more than 40 years adapting MT business processes to compensate for RbMT’s technical flaws, these compromises still influence most of today’s MT market.
Second Generation SMT
As I was learning about RbMT, academic researchers were honing a second generation MT technology called statistical machine translation (SMT). It borrowed technologies from speech recognition. These technologies performed at their best within narrow subject-constrained domains. SMT quickly found a home in unconstrained cloud-based applications serving millions of users.
To service millions of users from the Cloud, experts used huge translation memories (TMs) to expand SMT’s efficacy beyond narrow subject domains. This Big Data approach to SMT favored gist quality results across a broad range of subjects and sacrificed a high percent of correct results in favor of gist quality results across a broad range of subjects.
By 2007, corporate antibodies (https://www.youtube.com/watch?v=KGzXWO_anLI) forced the innovative SMT to conform to RbMT's business processes of complex error metrics and improvement cycles. After all, those metrics and processes were already in place. But, the underlying technical requirements had changed and our industry all-but forgot that SMT has the capacity to deliver correct results.
Now after 10 years of cloud-based Big Data SMT, Bigger is better has become a mantra but how does SMT with Big Data really perform?
In July this year, the private sector published a study showing the performance of cloud-based Big Data SMT across 25 language pairs for real-world professionals – Data: Machine and Professional Human Translations Identical in 5 – 20% cases. In 23 of the 25 pairs reported, less than 10% of the suggestions are good enough to use without change. Stated conversely, more than 90% of Google and Microsoft MT suggestions are not good enough to use without change.
Beyond the numbers, the good enough caveat might otherwise go unnoticed. In some cases, post-editors are encouraged to compromise their professional judgment to deliver less-than-correct translations.
Third Generation NMT?
There’s a third generation MT technology called Neural Machine Translation (NMT) gaining momentum. Slator.com (https://slator.com/technology/nearly-indistinguishable-from-human-translation-google-claims-breakthrough/) recently reported Google’s claim that NMT results are “nearly indistinguishable” from human translation. Only a week before, another Slator.com article (https://slator.com/academia/neural-machine-translation-improving-fast-study-finds/) reported an academic study that NMT reduced the overall post-editing effort 26% compared to SMT. As more of these reports take center stage in the media, let’s remember that a “26%” improvement over the 10% is good enough baseline. NMT is making progress, but it’s difficult to separate the hype from its promise.
What All This Means For memoQ Users
Keeping our feet on the ground and heads out of the clouds, a 10% good enough baseline sounds dismal. However for the first time, we have an objective reference to estimate a return on investment (ROI) for all memoQ’s supported MT providers.
This screen shot shows memoQ’s Machine translation options. Enabling a plugin will retrieve MT suggestions from MT providers including Google, Microsoft and others.
Let’s look at Google’s published pricing from the perspective of 10% good enough. When working in memoQ and the user clicks the new segment or, in other words, translation unit (TU), memoQ’s Google MT plugin sends the TU to Google and the user receives a suggestion. You can accept, edit or discard the suggestion. Regardless of whether you use the suggestion, you will pay 0.001¢ per character that the plugin sent to Google (US$ 20 per 1 million characters https://cloud.google.com/translate/v2/pricing).
We can calculate a rough annual cost of Google Translate using Google’s own estimated conversion rate (5¢ per page at 500 words per page is 0.01¢ per word). If you work at 2,000 words per day, 5 days per week and 50 weeks per year, your estimated yearly cost of Google Translate is US$ 50 per year. If we average that annual cost across the 10% good enough segments, your cost is 0.1¢ per good enough word.
¢ per word | 0.01 ¢ |
words per day | 2,000 |
days per week | 5 |
weeks per year | 50 |
words per year | 500,000 |
US$ per year | $50 |
good enough words @ 10% | 50,000 |
¢ per good enough word | 0.1 ¢ |
Engine Quality Evaluation
With the advent of SMT, MT technology entered the era of reliably generating a percentage of correct translations. Starting from a clean slate, we can create new metrics suited for SMT without the influences of the RbMT experience. These new metrics describe an engine’s ability to deliver correct translations, not the linguistic quality of each of its suggestions.
When working in memoQ, a TU with an edit-distance score of zero means you accepted the suggestion without changing it. In theory, 100% of the suggestions from an MT provider should score an edit-distance of zero. In reality, MT providers deliver significantly fewer than 100% correct suggestions. As the link above shows, Google and Microsoft on average deliver less than 10% correct.
In memoQ, you can measure an MT provider’s capacity to deliver correct suggestions separately and distinctly from the linguistic quality of the provider’s suggestions. This is where memoQ is at its best supporting MT.
- To evaluate memoQ’s MT providers, you need to setup a trail account with the related cloud-based service. Then, use memoQ’s analytics to monitor the provider’s edit-distance performance. Record the price per word and the actual edit distance zero rate (percent).
- Slate Desktop (SD) differs from the other providers because it’s not a cloud-based service. As a desktop application, SD converts your TMs into a translation engine..
Slate Desktop relies on SMT’s forgotten strength, i.e. it works best within narrow subject-constrained domains. Instead of using huge TMs, we recommend you use your translation memories to create personalized translation engines. Three or four years of your work in your TMs will prove that good things come in small packages.
Your zero-edit percent you experience when using the SD reflects the cumulative quality of your TMs. If your TMs consist of only your personal work, the suggestions will reflect your personal translation style. Current SD customers report zero-edit rates ranging from 30% to 50%, significantly higher than the 5% to 20% reported for cloud-based Google and Microsoft.
Other MT providers allow you to create custom engines from your TMs in the Cloud. These custom engines offer different features and may deliver comparable quality to SD using the same TMs.
Pricing Evaluation
Most memoQ’s MT providers except Slate Desktop offer subscription pricing. Their subscription prices vary but you can adjust the above calculations with your MT provider’s results to identify the MT provider that gives you the best value for your work.
SD’s pricing is a one-time perpetual license without subscriptions or other fees. You’ll need to adjust the above calculations to amortize or otherwise account for the one-time license fee. After expending the license fee, your price per word is zero.
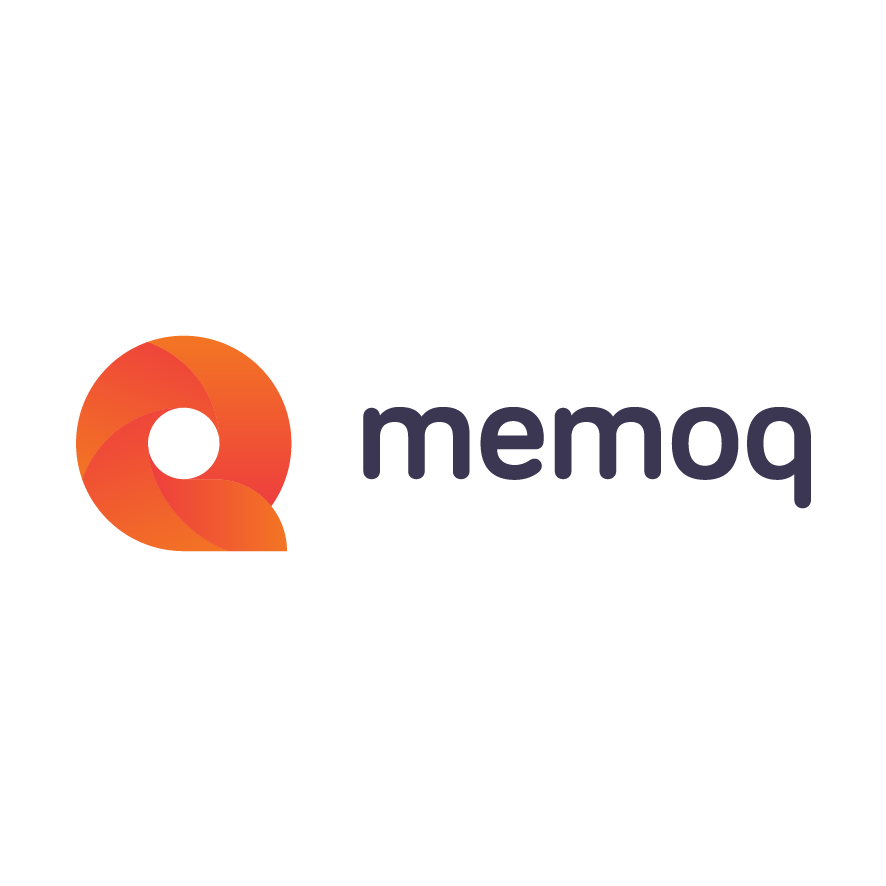
memoQ
memoQ is among the world's leading translation management systems. The favorite productivity tool for translation professionals around the globe.